Logan Ngai - Tevard Biosciences
- The Rivers School
- Aug 23, 2021
- 2 min read
This summer, I was blessed to have the opportunity to intern for Sean McFarland of Tevard Biosciences in Cambridge. Sean is a computational biologist who works primarily in Python, the computer language I learned and worked in this summer. Tevard Biosciences was founded by MIT molecular cell biologist Harvey Lodish, Daniel Fischer, and Warren Lammert, to combat Dravet syndrome and, eventually, other genetic diseases. More specifically, Dravet syndrome is a genetic mutation of the SCN1A gene on chromosome 2 that causes frequent seizures (epilepsy). Early stop codons that can result from random mutations can make the protein nonfunctional.
At the beginning of my internship, I focused more on the computational aspect of Tevard’s work with a focus on optimality and the secondary structure of proteins. I began by reviewing and learning more about the DNA transcription and translation processes and how mutations negatively impact the folding and optimization of proteins. Over the span of five weeks, I analyzed the human transcriptome and cataloged local optimality and secondary structure features that can be used to inform future modeling about how enhancers function.
I started my work on local optimality with just two things: nearly 20,000 mRNA sequences and the optimality of each codon. From here, with the help of Sean, I was able to derive the average and standard deviation of the optimality of the entirety of each transcript as well as minimum, maximum, and median averages for transcripts through a sliding window of codons size three through thirty.
I eventually moved on to looking at a few genes of interest, more specifically, SCN1A - the gene specific to Dravet syndrome. I used my findings and data from my general studies to compare the data with the SNC1A gene to look for any potential outliers that could be useful in future studies.
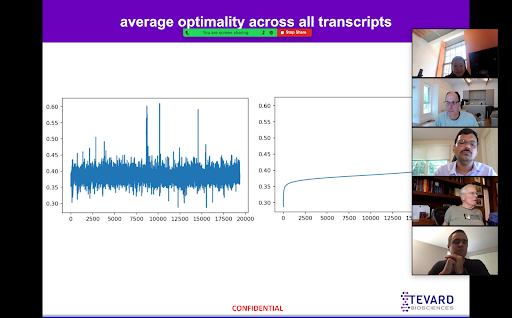
Next, I looked at the secondary structures of the proteins with the intent of looking for places of distinct optimality in strand, helix, and turn sequences. I began with XML files from the UniprotKB database and converted the information into a more accessible table by extracting the protein and gene name, the ensembl ID, amino acids, structure type, and the beginning and ending position of each structure type. From each structure, I found the optimality computationally, as well as the optimality of the codons before and after to look for any outliers. While there weren’t any distinct patterns in the data, future modelling of the enhancers should closely examine the role these features’ presence may play.
I am so grateful for the opportunity I had to work with Sean and all of Tevard Biosciences this summer. I have learned so much from meeting with the team members and learning about their experiences at the undergraduate and graduate level (and beyond) that will be invaluable as I move forward in the sciences. I look forward to working with Sean and other team members in the future and seeing how much good their work will do for so many young kids and families.
Comments